Translated by: Golden Finance
Note: On December 10, 2024, Crypto x AI project Hyperbolic announced the completion of a $12 million Series A financing, led by Variant and Polychain Capital. Together with Hyperbolic's previous $7 million seed round led by Polychain Capital and Lightspeed Faction, as well as earlier pre-seed financing, Hyperbolic's total financing amount reached $20 million.
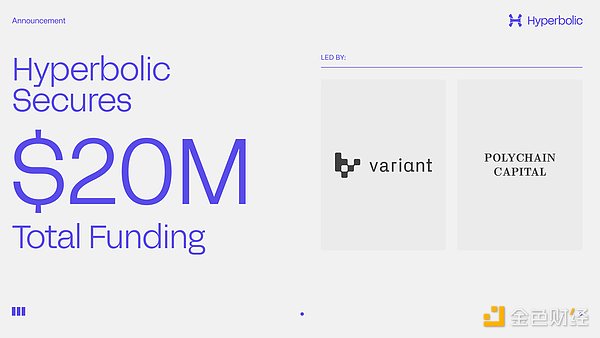
For what Hyperbolic is, please refer to Golden Finance's previous report "Understand Crypto x AI Rising Star Hyperbolic in One Article".
At the same time, Variant published an article on its official website to explain why it led the investment in Hyperbolic. Let's follow Variant to see Variant's investment logic for Hyperbolic.
The transformation of computing platforms often evolves in pairs or groups, usually accompanied by new hardware, applications, and distribution innovations, which drive each other forward. For example: PCs, the Internet, and the World Wide Web. Mobile devices, social networks, and cloud computing.
We believe a new powerful combination is emerging at the intersection of crypto and AI. AI requires large-scale coordination of GPUs, and crypto leverages incentives to pool resources. AI is probabilistic, while crypto is deterministic. We believe crypto can solve two of the most pressing problems facing AI: cost and trust (especially the cost of trust).
Cost and Trust
Let’s break this down:
Cost
It’s extremely expensive to run models right now. The root cause is often attributed to a supply problem, with the largest tech companies hoarding and causing GPU shortages. But that’s not the case; GPUs are plentiful in data centers, mining farms, PCs, and local machines around the world. Instead, GPUs appear scarce because their supply is fragmented and uncoordinated. So what we’re really facing is a coordination problem across a decentralized network of GPU suppliers, which makes them expensive.
Trust
Decentralized networks of GPUs have a lower raw cost, but they introduce a new problem: the cost of trust. How do you trust that a model run by a network of different participants is running correctly? The traditional solution in crypto is to have every node perform the same computation, introducing a lot of overhead, or to reduce the computational burden entirely.
But this doesn’t work for AI models, because having every node perform the same computation is too slow, and reducing the model size also reduces quality. There are undoubtedly verification problems in centralized environments as well (e.g., how do you know if ChatGPT gave you GPT-4o or GPT 3.5?), but OpenAI’s reputation can support trust more cheaply, despite lacking the rigor of cryptography. This verifiability may not matter when asking about cookie recipes, but it certainly matters when asking whether a malignancy is present in a medical image. As AI takes on more and more important roles in society, the cost of trust will only increase. Crypto networks are a step ahead in this regard, because they must solve the verification problem in order to reduce costs.
This is where Hyperbolic comes in.
What is Hyperbolic
Hyperbolic is the first player we’ve seen to solve the trust cost problem in decentralized GPU networks. A key innovation from the team that makes this possible is sampling-based verifiable machine learning (spML). It uses a random sampling protocol called Proof of Sampling to guarantee verifiability across a decentralized network of GPU providers (assuming the parties behave economically rationally) while maintaining the efficiency required to run the largest, highest quality AI models. Hyperbolic makes it possible to run models verifiably at a lower cost without sacrificing performance or quality.
Early market response supports this. Hyperbolic is one of only a few platforms that hosts the Llama 3.1 405B base model in BF16 format, a large open source model that is comparable in quality to OpenAI’s proprietary GPT-4o model, but running Llama 3.01 405B on Hyperbolic is 10x cheaper than using OpenAI’s GPT-4o model. Integrations with leading AI platforms such as Hugging Face’s Gradio, OpenRouter, and Quora’s Poe highlight Hyperbolic’s commitment to bringing the highest quality models to the AI community. Well-known AI developers like Andrej Karpathy have used Hyperbolic to run open source models because it is able to run higher quality models, more cheaply, and with a better user experience than competing products.
But Hyperbolic is more than just a formidable Web2 competitor, it will be unmatched in meeting the needs of Web3 applications. Currently, Web3 applications are forced to strike a Faustian bargain when integrating AI: in order to get the performance they need, they must rely on centralized sources of AI inference, which directly contradicts the decentralized ethos of the project and reintroduces the oracle problem. Because Hyperbolic will provide decentralization as well as performance and quality, Web3 applications will be able to take advantage of it without sacrificing either party.
We believe the team’s focus on first building a product that is competitive for all users, not just Web3 users, is the right approach. GPU supply is mercenary and will follow demand without hindrance, so it is critical to attract demand first to build the necessary stickiness. We expect that inference demand will continue to attract GPU supply and achieve the economies of scale required to compete in the market over the long term. A not-so-perfect analogy to explain this is Amazon's approach to AWS. Amazon first focused on building demand for compute through products that users love (such as its marketplace), providing the compute supply needed to support that demand, and ultimately achieving the economies of scale that enabled it to launch AWS and offer compute services to third parties cheaper and better than its competitors. After establishing core demand and supply on the Hyperbolic Network, we believe the team will be able to scale across all layers of the AI stack, including training, data sourcing, and preprocessing.
Hyperbolic Founding Team
Hyperbolic's founders are the strongest team we have encountered in this field,They have deep expertise in both encryption and artificial intelligence, which gives them a unique advantage in addressing the decentralized computing market for AI models.
In encryption,
Hyperbolic CEO and co-founder Jasper Zhangis a mathematics expert with expertise in proof verification of distributed systems.
Jasper has won awards in the Mathematical Olympiad many times, obtained a Ph.D. in mathematics from Berkeley in less than two years (becoming the fastest person to complete this five-year Ph.D. in the school's history), and was previously a quantitative analyst at Citadel and a researcher at Ava Labs.
In the field of encryption,
Hyperbolic CEO and co-founder Jasper Zhangis a mathematics expert with expertise in proof verification of distributed systems.
Jasper has won awards in the Mathematical Olympiad many times, and obtained a Ph.D. in mathematics from Berkeley in less than two years (becoming the fastest person to complete this five-year Ph.D. in the school's history).
On the AI side, Yuchen Jin, CTO and co-founder of Hyperbolic, is an expert in machine learning and distributed systems. Yuchen received the prestigious China National Scholarship, holds a PhD in Computer Science from the University of Washington, and manages a team of engineers at OctoAI building optimization solutions for AI models.
We are pleased to announce that we led Hyperbolic’s Series A round today. We are very excited to support Jasper, Yuchen, and the rest of the Hyperbolic team in their journey to make AI more accessible, verifiable, and open.