Source: AI Faner
Eight Google employees met by chance and co-wrote the groundbreaking "Converter" paper. This technological breakthrough completely changed the The field of artificial intelligence, especially in understanding and generating human-like text.
In the spring of 2017, a scientific paper titled "Attention Is All You Need" was published. The eight authors signed on it were all from Google, although one member had resigned at that time. When senior author Noam Shazeer saw the first draft, he was surprised to find that his name was listed first, which seemed to mean that his contribution was the most important. In this regard, he said: "I have not deliberately considered this issue."
In academia, how to arrange the names of authors has always been a delicate balance issue-whose name is placed first, whose And put it last. Especially in a situation like this where everyone leaves a unique mark in a true team effort. In the rush to complete the paper, the research team ultimately decided to break with convention and not rank contributors. They added an asterisk and a footnote next to each name: "Equal Contributor," and noted that "ranking order is random." The paper was subsequently presented to a prestigious artificial intelligence conference, where it sparked a revolution.
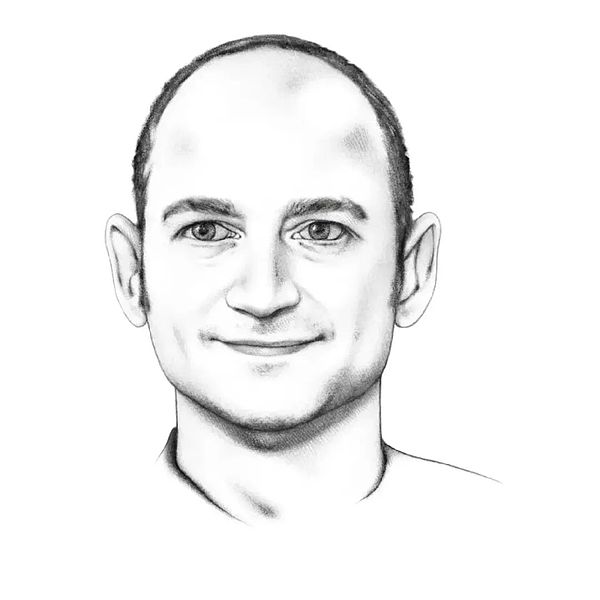
Name: NOAM SHAZEER / Occupation: Co-founder of Role AI & CEO
Now, as the Attention paper approaches its seventh anniversary, it has achieved legendary status. The authors of this paper started from a booming artificial intelligence technology - neural networks - and took it to a new level: they created a digital system so powerful that it seems to possess alien intelligence. This architecture, called "transformers," has become the mysterious power behind all the amazing AI products, including ChatGPT and the graph generators Dall-E and Midjourney.
Shazeer joked that if he had known how famous the paper would become, he "might have been more worried about the ordering of the author list." Today, all eight authors have become micro-celebrities. Llion Jones (randomly ranked fifth) said: "Someone asked me for a selfie because I once participated in a paper."

Name: LLION JONES/Occupation: Co-Founder of SAKANA AI
“Without the converter, I don’t think we would have today It will be here," said world-renowned AI scientist Geoffrey Hinton, although he was not an author of the paper. He's referring to the transformative era we live in, where companies like OpenAI are building systems that in some ways surpass even human output.
All eight of these authors later left Google. Now, they, like millions of others, are using the technology they created in 2017 in some way. I interviewed eight of the "Transformer" authors to try to piece together the full picture of this breakthrough—a collection of human ingenuity that created a machine that might ultimately end itself.
The story of the Converter begins with the fourth name on the list: Jakob Uszkoreit. His father, Hans Uszkoreit, is a well-known computational linguist. Hans was imprisoned in East Germany for 15 months in the late 1960s for protesting the Soviet invasion of Czechoslovakia. After his release from prison, he fled to West Germany and studied computers and linguistics in Berlin. He later came to the United States and worked at the SRI Institute in Menlo Park, California, around which time Jakob was born. Eventually, the family returned to Germany, where Jakob attended university.
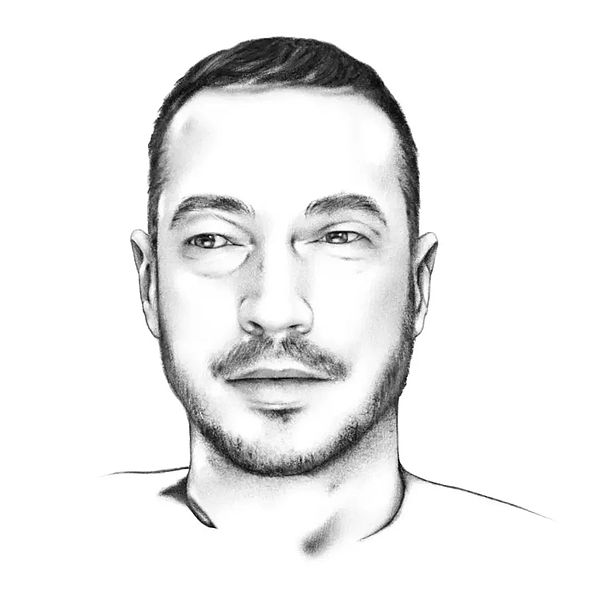
Name: JAKOB USZKOREIT / Occupation: Co-founder and CEO of INCEPTIVE CEO
Although he did not originally plan to focus on languages, when he began graduate school he interned at Google's Mountain View office and joined the company's translation team. He gave up his PhD plans and decided in 2012 to join a team at Google working on a system that could answer user questions directly on the search page, without redirecting users to other sites. At the time, Apple had just released Siri, a virtual assistant that promised to provide one-time answers in casual conversations, and Google executives thought Siri might threaten their search traffic. They began to pay more attention to Uszkoreit's new team.
"This is a false panic," Uszkoreit said. Siri doesn't really threaten Google. But he welcomes the opportunity to delve deeper into systems where computers talk to humans. At the time, recurrent neural networks—once a fringe area of academia—suddenly began to outpace other AI engineering methods. These networks are composed of multiple layers through which information is passed repeatedly to identify the best response.
Neural networks have achieved great success in areas such as image recognition, and the AI renaissance movement has suddenly emerged. Google is frantically adapting its workforce to adopt these technologies. Companies want to build systems that produce human-like responses—like automatically completing sentences in emails, or creating relatively simple customer service chatbots.
However, this field has encountered limitations. Recurrent neural networks have difficulty processing long blocks of text. For example, to understand "two hits" in the sentence "Joe is a baseball player, and after a good breakfast he went to the park and got two hits", the language model needs to remember information about baseball. In human terms, it has to stay focused.
The solution at the time was a technology called "long short-term memory" (LSTM), which allowed language models to process larger and more complex sequences of text. But the computer still processes these sequences strictly sequentially—word by word—and ignores contextual clues that might appear later in the text. "The approach we are applying is basically a stopgap measure," Uszkoreit said. "We couldn't really get the right stuff to work at scale."
Around 2014, he began to conceive of a different approach, which he called self-focus. This network can translate a word by referencing any other part of the text. These other parts can help clarify a word's intent and help the system produce a good translation. "It actually takes everything into account and gives you an efficient way to look at a lot of inputs at the same time and then take something out in a fairly selective way," he said. Although AI scientists are careful not to confuse neural network metaphors with how biological brains actually work, Uszkoreit seems to believe that self-focus bears some resemblance to the way humans process language.
Uszkoreit believes that self-attention models may be faster and more effective than recurrent neural networks. The way it processes information is also well suited to the mass-produced parallel processing chips that support the machine learning boom. Rather than taking a linear approach (looking at each word sequentially), it takes a more parallel approach (looking at multiple words simultaneously). If done correctly, Uszkoreit suspects, you can use self-focus exclusively to get better results.
Not everyone thought the idea would change the world, including Uszkoreit's father, who won two Google Faculty Research Awards while his son worked for the company. "People were skeptical about this because it throws away all existing neural architectures," says Jakob Uszkoreit. Say goodbye to recurrent neural networks? This is heresy! "My dad and I had conversations at the dinner table where we didn't exactly see eye to eye." Uszkoreit convinced some colleagues to experiment with self-focus. Their work showed promise and they published a paper about it in 2016. Uszkoreit wanted to push their research further—the team's experiments only used tiny portions of the text—but none of his collaborators were interested. Instead, like gamblers, they leave the casino with modest wins, applying the lessons they learned to various different areas of Google, including search and ultimately advertising. In many ways, it was a stunning success, but Uszkoreit didn't want to stop there.
Uszkoreit believes that self-focus can take on larger tasks. He would lay out his vision to anyone who would listen, and even some who wouldn't, sketching it out on a whiteboard in the building at 1945 Charleston Road on the north edge of Google's campus.
One day in 2016, Uszkoreit was having lunch with a scientist named Illia Polosukhin at the Google Cafe. Polosukhin, who was born in Ukraine, has worked at Google for nearly three years. He was assigned to a team that answered questions directly posed by the search domain. Things didn't exactly go well. "To answer something on Google.com, you need something very cheap and performant," Polosukhin said. "Because you only have milliseconds to respond." When Polosukhin expressed his complaint, Uszkoreit didn't hesitate to come up with a solution. "He suggested, why not use self-focus?" Polosukhin said.
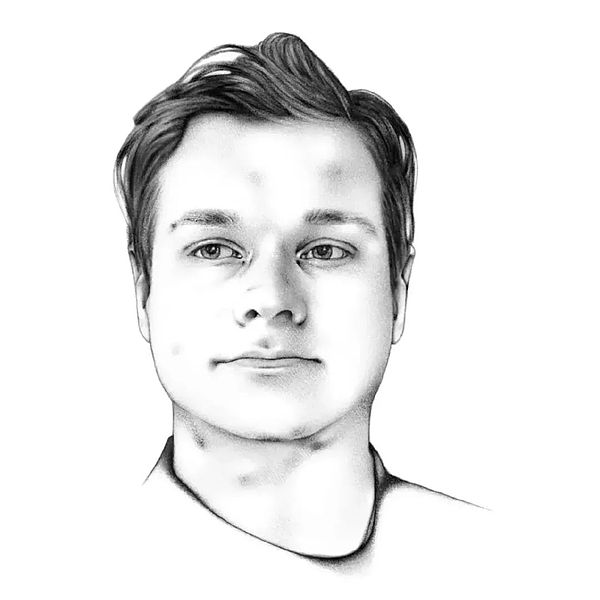
Name: ILLIA POLOSUKHIN/Occupation: Co-founder of NEAR< /strong>
Polosukhin sometimes collaborates with colleague Ashish Vaswani. Born in India and raised in the Middle East, Vaswani went to the University of Southern California to earn his PhD from an elite group in machine translation. Afterwards, he moved to Mountain View to join Google—specifically, a new organization called Google Brain. He describes the brain as "a radical team" and believes "neural networks will advance human understanding." But he was still looking for a big project to work on. His team worked next door to Building 1945, known as Building 1965, and he heard about the idea of self-focus. Would that be a project? He agreed to proceed.
The three researchers worked together to draft a design document titled "Transformers: Iterative Self-Focus and Processing on a Variety of Tasks." They chose the name "Transformer" from "day one," Uszkoreit said. The idea is that this mechanism will transform the information it receives, allowing the system to extract as much understanding as possible - or at least give the impression of it. Additionally, Uszkoreit has fond memories of playing with Hasbro action figure toys as a child. “I had two little Transformers toys when I was a kid,” he said. The document ends with a cartoonish image of six Transformers firing lasers at each other in a mountainous terrain.
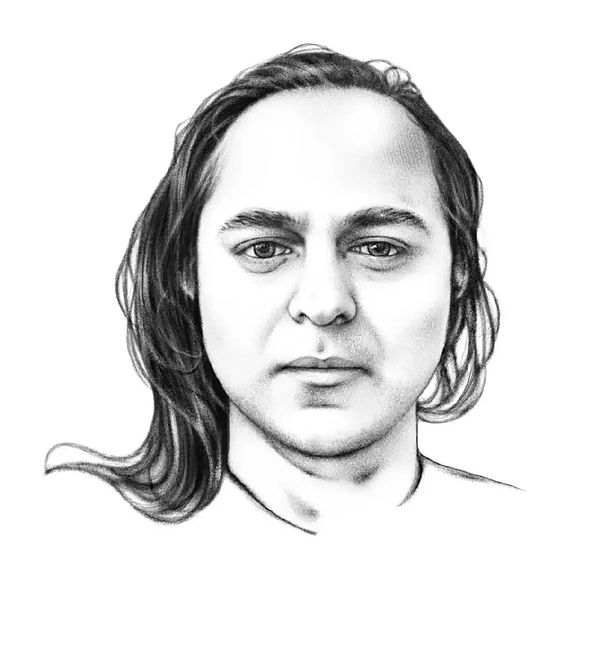
Name: ASHISH VASWANI/Occupation: Co-founder of ESENTIAL AI And CEO
The sentence at the beginning of the paper is also a bit arrogant: "We are great."
In early 2017, Polosukhin left Google to start his own company. By then, new collaborators had come on board. An Indian engineer named Niki Parmar worked in India for an American software company and later moved to the United States. She received her master's degree from USC in 2015 and was recruited by all the big tech companies. She chose Google. When she started working, she joined Uszkoreit and worked on improving model variations for Google Search.
Another new member is Llion Jones. Born and raised in Wales, he loves computers "because it's not normal". At the University of Birmingham, he took an AI course and became curious about neural networks as a historical relic. He earned his master's degree in July 2009 and lived on welfare for several months because he couldn't find a job during the recession. He found a job at a local company and then applied to Google as an "act of desperation." He got the job and ended up in Google Research, where his manager was Polosukhin.
One day, Jones heard about the concept of self-focus from a colleague named Mat Kelcey, who later joined the converter team. (Later, Jones met Kelcey and briefed him on the converter project. Kelcey wasn't buying it. "I told him, 'I'm not sure that's going to work,' which was basically the biggest misprediction of my life," Kelcey says now.)
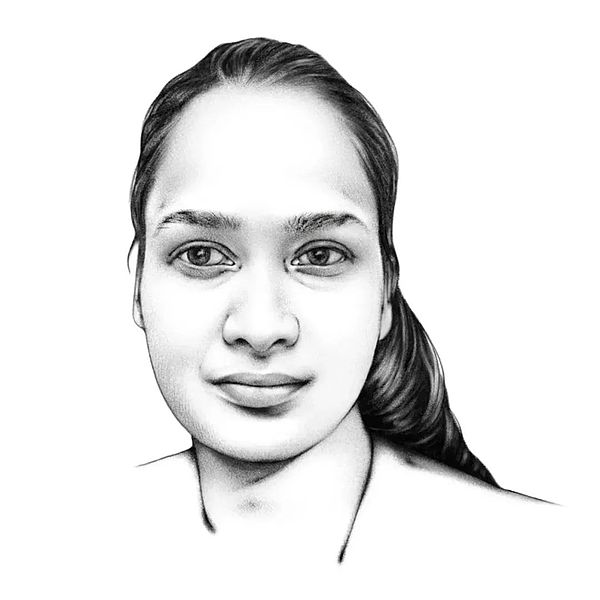
Name: NIKI PARMAR / Occupation: ESSENTIAL Co-founder of AI
Transformer's work has attracted other Google Brain researchers who are also trying to improve large language models. This third wave included Polish-born theoretical computer scientist Łukasz Kaiser and his intern Aidan Gomez. Gomez grew up in a small farming village in Ontario, Canada, where his family tapped maple trees every spring for maple syrup.
As a junior at the University of Toronto, he fell in love with AI at first sight and joined the machine learning group-Geoffrey Hinton's laboratory. He started contacting people who had written interesting papers at Google with ideas for extending their work. Kaiser took the bait and invited him to intern. It wasn’t until months later that Gomez learned these internships were for doctoral students, not undergraduates like himself.
Kaiser and Gomez soon realized that self-focus looked like a promising, more radical solution to the problem they were solving. "We consciously discussed whether we wanted to merge the two projects," Gomez said. The answer is yes.
The Transformer team started building a self-focused model to translate text from one language to another. They measured its performance using a benchmark called BLEU, which compares the machine's output to the work of human translators. From the start, their new model did a great job. “We went from having no proof of concept to having something that was at least comparable to the best alternatives to LSTM at the time,” Uszkoreit said. But compared to long-short-term memory, "it's not better."
They hit a plateau — until one day in 2017, Noam Shazeer happened to hear about their project. Shazeer is a veteran Googler -- he joined the company in 2000 -- and an internal legend, starting with his work on the company's early ad systems. Shazeer has been working on deep learning for five years and has recently become interested in large language models. But those models fell far short of producing the fluid conversations he thought were possible.
As Shazeer recalled, he was walking past Kaiser’s workspace in the hallway of Building 1965. He found himself listening to a lively discussion. "I remember Ashish was talking about the idea of using self-focus, and Niki was really excited about it. I thought, wow, that sounds like a great idea. This looks like a fun, smart team doing some promising things." Shazeer found existing recurrent neural networks "annoying" and thought: "Let's replace them!"
Shazeer's addition to the team was key. "These theoretical or intuitive mechanisms, such as self-focus, always require very careful implementation, usually by a small number of experienced 'magicians' to show any signs of life," Uszkoreit said. Shazeer immediately started working his magic. He decided to write his own version of the Transformer team's code. “I took the basic idea and made it myself,” he said.
Occasionally he would ask Kaiser a question, but mostly, he said, he "just worked on it for a while and then came back and said, 'Look, it worked.'" Using "Magic" ”, “alchemy” and “bells and whistles” to describe something he took the system to the next level.
"That sparked a rush," Gomez said. They are motivated, and they also want to meet the upcoming deadline of May 19 to publish papers at the largest AI event of the year, the Neural Information Processing Systems conference in December. Submission date. As winter turns to spring in Silicon Valley, the pace of experimentation accelerates. They tested two transformer models: one produced with 12 hours of training, and a more powerful version, called Big, trained for three and a half days. They got them started on English to German translation.
The base model outperformed all competitors - and Big achieved a BLEU score that decisively beat the previous record while being computationally more efficient. "We did it, faster than anyone," Parmar said. “And that’s just the beginning, because the numbers keep improving.” When Uszkoreit heard the news, he celebrated by taking out a bottle of old champagne he kept in his mountain adventure truck.
In the last two weeks before the deadline, the team worked at a frantic pace. Although some team members officially still have desks in Building 1945, they mostly work in Building 1965 because there is a better espresso machine in the micro kitchen. “People barely slept,” recalls Gomez, who, as an intern, was busy debugging while also producing the paper’s visualizations and diagrams. In such projects, ablation experiments are often performed—removing certain parts to verify that the remaining parts are sufficient to complete the task.
“We tried every possible combination of tricks and modules—what worked and what didn’t. We were constantly trying and replacing,” Gomez said. "Why does the model behave in this counterintuitive way? Oh, because we forgot to do the masking correctly. Now does it work? Okay, so on to the next one. All these components we now call transformers It's all a product of this high-speed, iterative trial-and-error process." With the help of Shazeer's implementation of the code, the ablation experiments produced "somewhat parsimonious results," Jones commented. "Noam is a wizard."
Vaswani remembers spending the night on the office couch while the team was working on a paper. He stared at the curtain that separated the couch from the rest of the room, drawn to the patterns on it that looked like synapses and neurons. Gomez was there, and Vaswani told him that the work they were doing would go beyond machine translation. “Ultimately, just like the human brain, you need to unify all these modalities — speech, audio, visual — under a single architecture,” he said. "I had a strong hunch we were discovering something more general." At the top of Google, however, the work was viewed as just another interesting AI project. The authors were asked whether their supervisors frequently called them in to update them on project progress, and the answers were modest. But "we know this could be a pretty big thing," Uszkoreit said. "That led to us actually becoming obsessed with a sentence at the end of the paper."
That sentence foreshadowed what might come next—the application of the transformer model to essentially all forms of human expression. "We are excited about the future of attention-based models," they wrote. "We plan to extend the transformer to problems involving input and output modalities other than text" and study "images, audio, and video."
One evening a few days before the deadline, Uszkoreit Realized they needed a title. Jones points out that the team has made a fundamental rejection of one technology: attention. The Beatles once titled a song "All You Need Is Love." Why not name the paper "Attention Is All You Need"?
"I'm British," Jones said. "It really only took five seconds of thinking. I didn't expect them to use it."
They continued to collect the results of the experiment until the deadline. "Five minutes before we turned in the paper, the English and French numbers came out," Parmar said. "I was sitting in the micro kitchen in Building 1965 and got the last number." They had only two minutes left and rushed to send it. thesis.
Google, like nearly every other technology company, quickly filed for a provisional patent on the work. The reason is not to prevent others from using the ideas, but to build up their patent portfolio for defensive purposes. (The company's philosophy is "If technology advances, Google will reap the benefits.")
When the Transformer team heard feedback from the conference's peer reviewers, the reaction was mixed. "One is positive, one is extremely positive, and one is, 'This is OK,'" Parmar said. Papers were accepted for presentation in the evening poster session.
By December, the paper began to cause a stir. Their four-hour meeting on December 6 was packed with scientists who wanted to learn more. Authors talk about it until their voices get hoarse. At 10:30 pm, when the meeting ended, there was still a group of people. "The security had to tell us to leave," Uszkoreit said. Perhaps the most satisfying moment for him was when computer scientist Sepp Hochreiter came up to praise the work—quite a compliment considering that Hochreiter was the co-inventor of long-short-term memory, which the converter had just introduced. Replaced as the tool of choice in the AI toolbox.
Changers didn’t immediately take over the world, or even Google. Kaiser recalled that around the time the paper was published, Shazeer proposed to Google executives that the company should abandon its entire search index and train a huge network with transformers—basically, using transformers to change the way Google organizes information. At the time, even Kaiser thought the idea was ridiculous. Now, conventional wisdom says it's just a matter of time.
A startup called OpenAI seized on the opportunity faster. Shortly after the paper was published, OpenAI's principal researcher Ilya Sutskever—who knew the Transformer team from his days at Google—suggested that its scientist Alex Radford look into the idea. The result was the first GPT products. As OpenAI CEO Sam Altman told me last year, "When the transformer paper came out, I don't think anyone at Google realized the significance of it." Internally, the situation is more complicated. "It's clear to us that converters can do truly amazing things," Uszkoreit said. "Now, you might ask, why didn't Google launch ChatGPT in 2018? Realistically, we could have had GPT-3 or even 3.5 in 2019, maybe 2020. The real question is not, did they see it? The question is, Why aren't we doing anything with the facts we've seen? The answer is complicated."
Many tech critics point to Google's transformation from an innovation-focused playground to a bottom-line-focused bureaucracy mechanism. As Gomez told the Financial Times, "They didn't modernize. They didn't adopt the technology." But for a giant company whose technology has led the industry for decades and made huge profits, this takes a lot of guts. Google did start integrating translators into products in 2018, starting with its translation tools. In the same year, it introduced a new transformer-based language model, BERT, which was applied to search the following year.
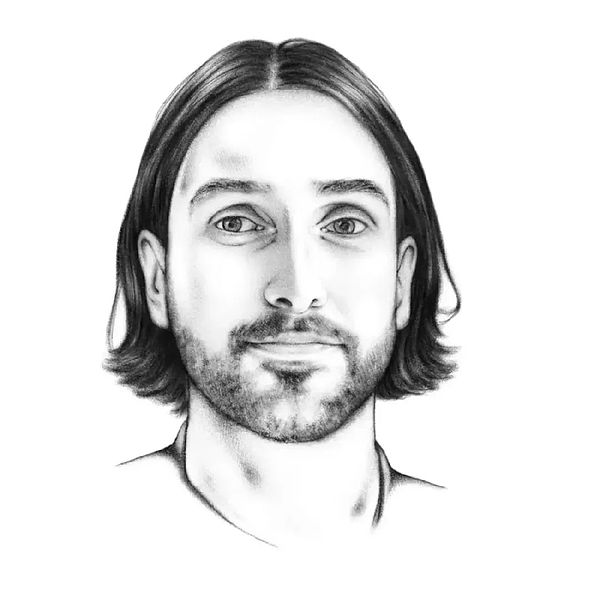
Name: AIDAN GOMEZ/Occupation: Co-founder and CEO of COHERE CEO
But these behind-the-scenes changes seem timid compared to OpenAI's leaps and Microsoft's bold integration of transformer-based systems into its product line. When I asked CEO Sundar Pichai last year why his company wasn't the first to launch a large language model like ChatGPT, he argued that in this case, Google found it advantageous to let others take the lead. "I'm not quite sure yet if it's going to be as successful as it is. The fact is, once people see how it works, we can do a lot more," he said.
It is undeniable that all eight authors of the paper have left Google. Polosukhin's company, Near, built a blockchain whose tokens have a market capitalization of about $4 billion. Parmar and Vaswani became business partners in 2021, co-founded Adept (valued at $1 billion) and are now running their second company called Essential AI ($8 million in investment).
Tokyo-based Llion Jones’ Sakana AI is valued at $200 million. After Shazeer left in October 2021, he co-founded Character AI (valued at $5 billion). Intern Aidan Gomez co-founded Toronto-based Cohere (valued at $2.2 billion) in 2019. Jakob Uszkoreit’s biotech company Inceptive is valued at $300 million. All these companies (except Near) are based on converter technology.
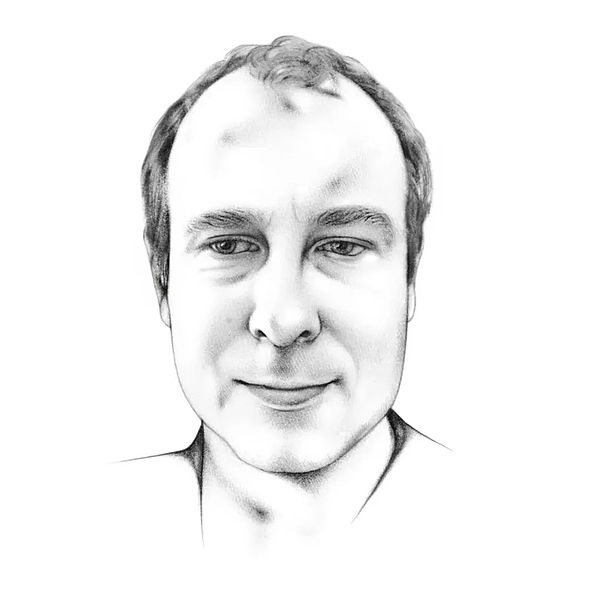
Name: LUKASZ KAISER / Occupation: Researcher at OPENAI
Kaiser is the only one who has not founded a company. He joined OpenAI and became the inventor of a new technology called Q*, which Altman said last year would "push the veil of ignorance and push the frontier of discovery forward." (When I tried to explain this in our When Kaiser was asked about this in an interview, OpenAI's publicist nearly jumped over the table to stop him.)
Does Google miss these deserters? Except, of course, others are moving from companies to new AI startups. (Pichai reminded me, when I asked him about Transformer’s departure, that industry darling OpenAI has also seen defections: “The AI space is very, very dynamic,” he said.) But what Google can boast is that it has created a platform to support An environment to pursue unconventional ideas. "In many ways, Google has been ahead of the curve - they invested in the right minds and created an environment where we can explore and push the limits," Parmar said. "It's not surprising that it took time to get adopted. Google had more at stake."
Without that environment: there would be no converter. Not only are the authors Googlers, they also work in the same office. Chance encounters in the hallways and small talk over lunch led to big moments. The team is also culturally diverse. Six of the eight authors were born outside the United States; the others are the children of two Germans with green cards who are temporarily in California, and an American whose family fled persecution for a generation.
Uszkoreit said from his office in Berlin that innovation is all about the right conditions. "It's about bringing together people who are really excited about something at the right time in their lives," he said. "If you have this, and you have fun doing it, and you're dealing with the right problems - and you're lucky - magic happens."
There is also a relationship between Uszkoreit and his famous father Something magical happened. After all those dinner table debates, Hans Uszkoreit, his son reports, has now co-founded a company that is building large language models. Of course, a converter is used.