Allora is an open-source, permissionless intelligence marketplace.
Allora aggregates
Consumers pay to acquire inferences or expertise that need to be revealed
Workers who reveal inferences
After the truth is revealed, Evaluators decide how accurate the workers are
Validators who ensure the protocol state, history, and reward distribution
With these elements, Allora is able to continuously improve itself over time and produce inferences that are more accurate than the most accurate participant.
The Allora adapter is currently running on Sepolia.
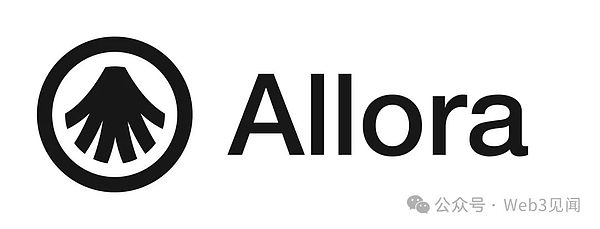
The Allora network is a network of machine learning (ML) models that are incentivized to promote the collective optimization of some underlying objective function. The machine intelligence network is composed of multiple sub-networks, each defined by its own objective function, ranging from predicting the price of an asset at some time in the future to constructing a portfolio to optimize some risk/return profile. This creates a network with greater applicability than the capabilities of its individual subnetworks.
Machine learning and artificial intelligence (AI) play an important role in many contexts. They leverage massive computational power and data-driven algorithms to discover insights, make predictions, and drive optimizations that are beyond the scope of human cognition or currently expressed on-chain. Machine intelligence networks allow us to harness this power in a decentralized form, allowing us to build more advanced on-chain primitives.
In the case of Allora, AI-driven decentralized finance (DeFi) is its most immediately useful primitive. The network is optimized for financial applications and incentivizes network participants to contribute their asymmetric insights into financial markets (i.e., their “alpha”). Network participants submit their alpha in the form of data, predictive model features, and predictions, ultimately with the goal of improving Allora.
An Alpha Proof-of-Stake mechanism then aggregates and rewards network participants based on the usefulness of their contributions to optimizing certain objective functions, such as minimizing mean absolute directional loss or maximizing the Sharpe ratio. Additional security is built into any application built on the network in the form of verifiable computation. This is achieved through a new zkSNARK proof system designed to verify the output of tree-based ML models. Leveraging this proof system is a new tool for predicting asset prices using ML models, which we call zkPredictor. It was built in collaboration with and powered by Modulus Labs. The result is a decentralized, self-improving smart network optimized for modeling financial markets.