Source: Zeping Macro
On February 16, OpenAI released the video generation model Sora, which greatly expanded AI's capabilities in video content generation. Sora is significantly ahead of some previous video generation models in key indicators. If you use it to generate videos, you will find that its spatial simulation capabilities of the physical world have even reached a level that is close to reality.
Why can Sora be called a new milestone in the AI industry? How does it break through AIGC, the upper limit of AI content creation? Objectively speaking, are there any limitations or shortcomings in the current version of Sora?
What is the direction of future updates and iterations of video generation models such as Sora? Which industries will its emergence disrupt? What impact does it have on each of us? What new industry opportunities are behind it?
1. How is Sora implemented? Why is it a new milestone in the AI industry?
The reason why Sora is an AI milestone is that it once again breaks through the upper limit of AIGC's AI-driven content creation. Previously, everyone has begun to use text-based content creation such as Chatgpt to assist with illustration and screen generation, and use virtual humans to make short videos. Sora is a large model for video generation. Videos can be generated, connected, extended and edited by inputting text or pictures. It belongs to the category of multi-modal large models. This type of model is a further extension of large language models such as GPT. ,expand. Sora handles video "patches" in a manner similar to how GPT-4 operates on text tokens. The key innovation of this model is to treat video frames as sequences of patches, similar to word tokens in language models, allowing it to effectively manage a variety of videos. This approach, combined with text-conditioned generation, enables Sora to generate contextually relevant and visually coherent videos based on textual cues.
In principle, Sora mainly implements video training through three steps. The first is the video compression network, which reduces the dimensionality of videos or pictures into a compact and efficient form. The second is spatio-temporal patch extraction, which decomposes the view information into smaller units. Each unit contains a part of the spatial and temporal information in the view, so that Sora can perform targeted tasks in subsequent steps. deal with. The last step is video generation. Text or pictures are input for decoding and encoding. The Transformer model (i.e., ChatGPT basic converter) determines how to convert or combine these units to form a complete content in the text and picture prompts. video.
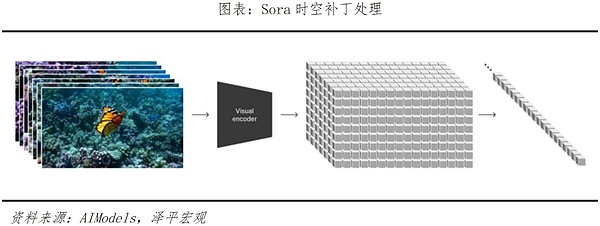
Sora’s two most critical indicators in the video generation model—— It greatly surpasses previous models in terms of duration and resolution, and has strong text understanding depth and detail generation capabilities. It can be said to be another milestone product in the AI industry. Before the release of Sora, the generation time of major models such as Pika1.0, Emu Video, and Gen-2 were 3~7 seconds, 4 seconds, and 4~16 seconds respectively; while Sora can generate times as long as 60 seconds, which can be achieved 1080p resolution, and Soracan not only generate videos based on text prompts, but also has video editing and expansion capabilities. Sora also has a strong in-depth understanding of text. With extensive text parsing training, Sora can accurately capture and understand the emotional meaning behind text instructions, and smoothly and naturally transform text prompts into detailed and scene-matching video content.
Sora can better simulate the physical laws of a virtual world in video generation, better understand the physical world, and produce a realistic lens feel. Its technical features mainly include two:
First, it can generate coherent three-dimensional space motion videos from multiple lenses.
The second is to maintain the consistency of the same object under different viewing angles. In this way, the model can maintain the coherence and persistence of the movement of characters, objects, and scenes in the video, and can affect elements in the world through fine-tuning and perform simple interactions. Compared with previous models such as Pika, Sora's generated video can also accurately understand elements such as video color style and create video content with rich facial expressions and vivid emotions. It also pays attention to the relationship between the subject and the background, making the interaction between the video subject and the background highly smooth and stable, and the storyboard switching is logical.
In an example of the generated video given by the official: "A fashionable woman walks on the streets of Tokyo, which are full of warm-toned neon lights and animated city signs. She is wearing black Leather jacket, long red skirt and black boots, holding a black leather bag. She wore sunglasses and red lipstick. She walked confidently and casually. The street was wet and reflective, creating a mirror effect with the colorful lights. Many pedestrians walked "Back and forth",Sora achieves a completely detailed description, even down to the description of skin details, and is realistic in the processing of details such as light and shadow reflection movement, lens movement, etc.
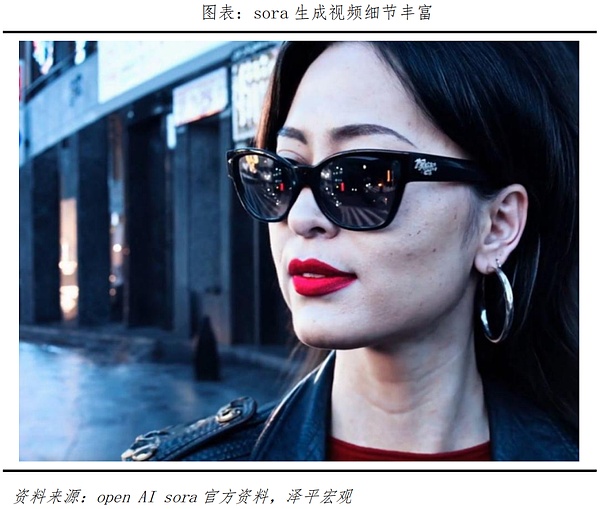
2. What level is Sora at? What are the limitations?
Sora is equivalent to ChatGPT3.5 of language model. It is a major breakthrough in the industry and is at a very leading level, but it still has its own limitations.
Sora and ChatGPT have the same origin as the Transformer architecture. The former builds a diffusion model based on the architecture, which is excellent at displaying depth, object permanence and natural dynamics. Previous real-world simulations were usually run using GPU-driven game engines for 3D physical modeling, which required manual construction and complicated processes with high accuracy to achieve high-standard environment simulations and various interactive actions. However, the Sora model does not have a data-driven physics engine and graphics programming, and its accuracy is low in higher-demand three-dimensional construction. Therefore, achieving a natural interaction of multiple characters and realistic simulation with the environment remains difficult.
For example, here are two examples of bugs in Sora-generated videos:
When Sora inputs the text "An overturned glass spilled liquid." ”, the glass is shown melting into the table, with the liquid jumping across the glass, but without any glass-shattering effect.
For another example, a chair is suddenly dug out from the beach, and the AI thinks that the chair is an extremely light material that can float directly.
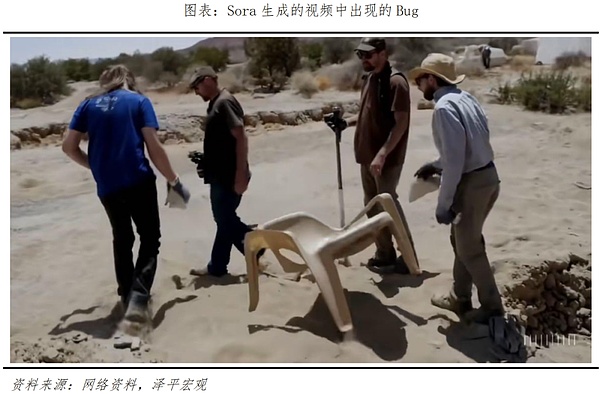
There are two main reasons for such "errors":
First, because the model is automatically completing the generated content, it spontaneously generates objects or entities that are not included in the text plan. This situation is particularly common, especially Is in a crowded or cluttered scene. In some scenarios, this will increase the realism of the video, such as in the case of "Walking on the streets of Japan in winter" given by OpenAI, but in more environments it will reduce the rationality of physical laws in the video, such as The table generated out of thin air in the first example is made of water.
The second is that when many actions occur in Sora's simulation, it is easy to confuse the order, including temporal order and spatial order. For example, when typing "person running on a treadmill" it has a chance of generating a person walking on a treadmill in the wrong direction. Therefore, Sora accurately simulates more complex real-world physical interactions, dynamics, and causal relationships, and it is still challenging to simulate simple physics and object properties.
Despite these ongoing issues, Sora demonstrates the future potential of video models. Given enough data and computing power, video converters may begin to gain a deeper understanding of real-world physics. ,Causal relationship. This may enable new methods of training AI systems based on video simulations of the world.
3. What challenges and opportunities does Sora face in its development direction?
Sora represents the forefront of video generation AI, but its future performance improvements may come from three major directions:
The first is to start from the data dimension. With the surge in data demand for training, we will face the problem of lack of trainable data samples in the future. Currently, the main large models rely on language text. Although Sora can also perform image input, the training breadth is not as good as text. The data types are single and high-quality data is limited, which may be quickly exhausted in the context of exponential increases in parameter volume.
Cornell University research shows that high-quality data for large model training is likely to be exhausted before 2026, and low-quality text data will be exhausted after 2030. Expanding the dimensions of data sources is Sora's solution. In addition to text and images, audio, video, thermal energy, potential energy, and depth can all become expansion areas for Sora learning. Help it become a truly multimodal large model. For example, Meta's open source ImageBind has multiple senses. It not only has the image and video recognition capabilities of DINOv2, but also has infrared radiation and inertial measurement units, which can sense and learn different modes such as depth, thermal energy, and potential energy. After Sora expands the input end, it can also better combine the above dimensions with video generation to train and simulate a more realistic physical world.
The second is to optimize from the algorithm level. Solving the "over-fitting" and "under-fitting" phenomena that exist in model learning is the key. As mentioned in the previous example, Sora will spontaneously generate objects or entities that are not included in the text plan, which helps to improve the authenticity of the video effect. However, in some cases, two highly correlated elements may appear at the same time in inapplicable scenarios, that is, the algorithm "overfits" in order to achieve a specific result. This phenomenon is similar to how humans repeatedly and intensively train to answer a type of questions correctly during exam preparation, but instead lead to a large number of errors in similar questions in the exam.
In the same example, the cup was knocked over but melted without breaking. This is because the model is "underfitting". The reason for these two types of problems in the model is that samples that are not accurately classified are selected for training, and the decision tree formed is not an optimal model, resulting in a decline in the generalization performance of real applications. Overfitting and underfitting cannot be completely eliminated, but they can be alleviated and reduced through some methods in the future, such as: regularization, data cleaning, reducing the size of training samples, Dropout abandonment, pruning algorithms, etc.
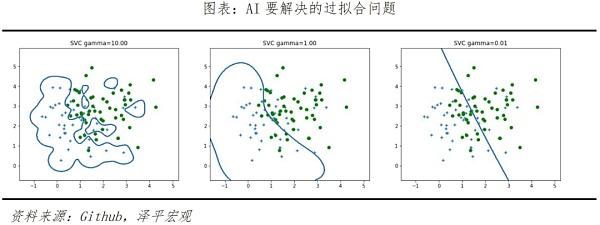
The third is the computing power industry. Sora continues to detonate the AI wave, which will also lead to the demand for computing power in 2024 to continue to rise under the development of multi-modal models. AI companies seek greater upstream entry into the industry chain, to chip R&D and design layout, and even to EDA and wafers. Advance in the field.
Currently, AI model training mainly relies on NVIDIA GPUs, but mainstream computing power chips are already in short supply, and demand is predicted to reach 1.5-2 million by 2024.
OpenAI founder Sam Altman has been paying attention to the supply and demand issue of his chips since 2018. He invested in the AI chip company Rain Neuromorphics and purchased Rain’s chips in 2019. In November 2023, Sam started working for a company code-named " Tigris' chip company seeks billions of dollars in financing. As an industry leader, it has already made early plans to build a computing power industry chain led by itself, aiming to reshape the global semiconductor landscape through the AI industry revolution.
Tesla, which has entered the AI track with smart cars, is also moving towards upstream chip design based on the basics of autonomous driving algorithms, and is gradually seeking to control the midstream. .
It is foreseeable that although the global AI semiconductor industry chain built by ARM, Nvidia, and TSMC is the biggest beneficiary in the short term, it may usher in greater competition in the medium and long term. The independent construction of computing infrastructure, especially computing chips, is still an important direction for China to keep pace with the world on the AI track.
4. Which industries will Sora’s application fields subvert?
From the release of Apple’s Vision Pro head-mounted display device at the beginning of the year, to the successive releases of AIPC by major PC manufacturers, to the release of Sora by OpenAI this time, the world has become more concerned about artificial intelligence. Innovation is accelerating, and iteration is getting faster and faster.
In the future, content automatically created with AI will affect many industry fields. "Timely coverage" of hot topics will mainly be the task of AI, and the main competition will be AIGC The efficiency of the competition is everyone’s ability to control AI, and the competition is about who can control a powerful AI production tool like Sora. In the future, it will not be impossible to "throw out a novel and produce a blockbuster". Sora can generate a video of up to 1 minute. The video can be shot to the end, switched between multiple angles, and the object remains unchanged. Sora videos can also use lens language such as scenery, expressions and colors to express emotional colors such as loneliness, prosperity, and cuteness. In short, if more Sora appears in the future, or if these large video-generating models undergo more improvements and lags from the above-mentioned angles, the future AI video effects may be almost as good as manual shooting.
The application of multi-modal models will usher in the dawn of 2024, affecting several industries such as film and television, live broadcast, media, advertising, animation, and art design. In the current short video era, Sora "one person" takes care of all tasks such as photography, directing, and editing of short videos. In the future, the various videos generated by Sora for different purposes will have a profound impact on the current short video, live broadcast, film and television, animation, advertising and other industries.
For example, in the field of short video creation, Sora is expected to greatly reduce the overall cost of short play production and solve the common problem of "emphasis on production but not on creation". The focus is expected to return to high-quality script content creation in the future, which will test the creative ability of outstanding creators. Sora is expected to truly reduce costs and increase efficiency for companies in related industries. Advertising production companies use Sora models to generate advertising videos that match the brand, significantly reducing shooting and post-production costs; game and animation companies use Sora to directly generate games Scene and character animation, reducing 3D model and animation production costs. The costs saved by enterprises can be used to improve product and service quality or technological innovation to further improve productivity. If 2023 is the explosion of global AI large models and the first year of image and text generation, then 2024 will be the year when the industry enters the first year of AI video generation and multi-modal large models. From Chatgpt to Sora, the real impact and changes of AI on every individual and every industry are gradually taking place.