Author: Teng Yan, Chain of Thought; Translation: Golden Finance xiaozou
I have a big regret that still haunts me today, it was undoubtedly the most obvious investment opportunity for anyone who paid attention to it, but I didn't invest a penny. No, I'm not talking about the next Solana killer, nor the dog meme coin with a funny hat.
It's...NVIDIA.
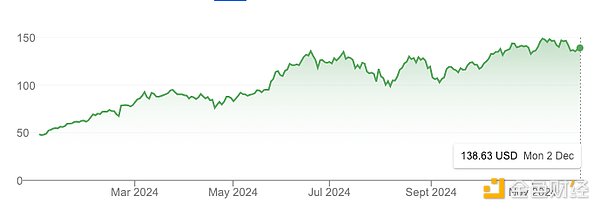
In just one year, NVDA's market value soared from $1 trillion to $3 trillion, a 3-fold increase, even surpassing Bitcoin in the same period.
Of course, there is artificial intelligence hype, but a large part of it is based on reality. NVIDIA announced its revenue of $60 billion in fiscal 2024, an amazing 126% increase from fiscal 2023.
So why did I miss it?
For two years, I was so focused on crypto that I didn’t look outside the box and didn’t pay attention to the field of artificial intelligence. I made a huge mistake that still haunts me.
But I won’t make the same mistake again.
Today, Crypto AI feels very similar. We are on the verge of a huge explosion of innovation. It’s too similar to the California Gold Rush in the mid-1800s to ignore—industries and cities are emerging overnight, infrastructure is developing at a breakneck pace, and wealth is being created by those who dare to think and do.
Like NVIDIA in its early days, Crypto AI will be an opportunity that is obvious in hindsight.
In the first part of this article, I will explain why Crypto AI is the most exciting underdog opportunity for investors and builders today.
A quick overview:
Many still think it’s fantasy.
Crypto AI is still in its early stages and may be 1-2 years away from the peak of hype.
This field has at least $230 billion+ growth opportunity.
In essence, Crypto AI is AI built on crypto infrastructure. This means it is more likely to follow the exponential growth trajectory of AI than the broader crypto market. Therefore, in order to keep up with the latest AI research on Arxiv and talk to founders who believe they are creating the next amazing products and services.
In the second part of this article, I will delve into four of the most promising sub-areas in Crypto AI:
Decentralized Computing: Training, Inference, and the GPU Market
Data Networks
Verifiable AI
AI Agents Running on Chain
This article is the culmination of several weeks of in-depth research and conversations with founders and teams in the Crypto AI space. This article is not intended to be an exhaustive dive into each area, but rather, you can think of it as a high-level roadmap designed to spark your curiosity, improve your research, and guide your investment thinking.
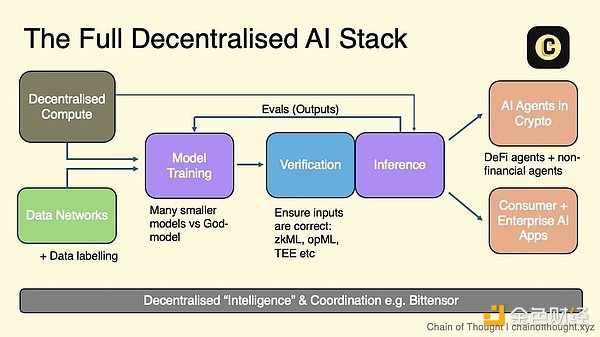
1. Crypto AI Landscape
I portray the decentralized AI stack as an ecosystem with several layers: one end starts with decentralized computing and open data networks to support decentralized AI model training.
Then, cryptography, crypto-economic incentives, and evaluation networks are combined to verify each inference—both input and output. These verified outputs flow to AI agents that can run autonomously on the chain, as well as consumer and enterprise AI applications that users can truly trust.
The coordination network ties everything together, enabling seamless communication and collaboration across the ecosystem.
In this vision, anyone building AI can leverage one or more layers of this stack, depending on their specific needs. Whether leveraging decentralized compute for model training or using evaluation networks to ensure high-quality output, the stack offers a range of options.
Due to the inherent composability of blockchains, I believe we will naturally move towards a modular future. Each layer is becoming highly specialized, with protocols optimized for different functions, rather than an all-in-one integrated approach.
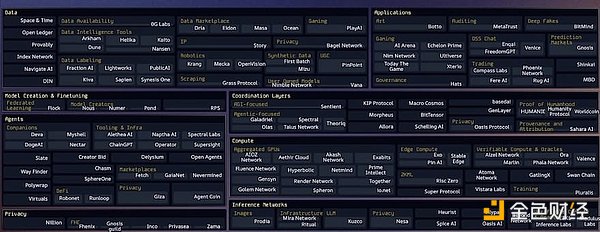
There is a large number of startups at each layer of the decentralized AI stack, most of which were founded in the past 1-3 years. It is clear that the field is still in its early stages.
The most comprehensive and up-to-date Crypto AI startup map I have seen is maintained by Casey and her team at topology.vc. This is an invaluable resource for anyone tracking the field.
As I delved deeper into the Crypto AI subfield, I kept asking myself: How big is the opportunity? I’m not interested in small-time bets—I’m looking for markets that are hundreds of billions of dollars in size.
(1) Market size
Let’s look at market size first. When evaluating a segment, I ask myself: Is it creating an entirely new market or disrupting an existing one?
Take decentralized computing as an example. This is a disruptive category whose potential can be assessed by looking at the existing cloud computing market, which is currently valued at around $680 billion and is expected to reach $2.5 trillion by 2032.
New markets that have never been seen before, such as artificial intelligence agents, are more difficult to quantify. In the absence of historical data, their evaluation requires guesswork and an assessment of the problems they are solving. It’s important to note that sometimes, what looks like a new market is actually just a solution in search of a problem.
(2) Timing
Timing is everything. Technology tends to improve and become cheaper over time, but the pace of improvement varies.
How mature is the technology in a particular niche? Is it ready for large-scale adoption, or is it still in the research phase, with real-world applications still years away? Timing determines whether an industry deserves immediate attention or a “wait and see” approach.
Take fully homomorphic encryption (FHE) as an example: its potential is undeniable, but its current pace of development is still too slow to be widely used. It may be several years before we see mainstream adoption. By focusing first on areas that are closer to scale, I can spend my time and energy on areas that are gathering momentum and opportunity.
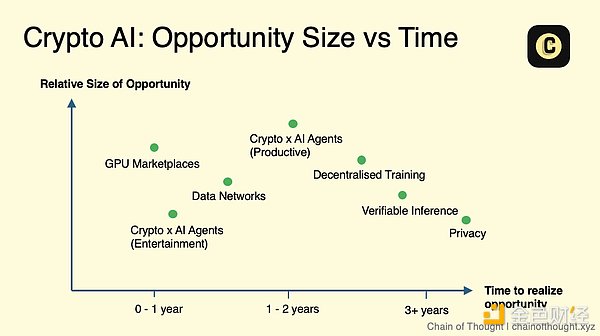
If I were to map these categories onto a scale vs. time chart, it would look something like this. Keep in mind that this is still a conceptual chart, not a hard and fast guide. There are a lot of nuances — for example, different approaches like zkML and opML have different levels of readiness for use in verifiable reasoning.
That said, I believe AI will scale so large that even areas that seem “niche” today could evolve into a significant market.
It’s also worth noting that technological progress doesn’t always follow a straight line — it often moves in leaps and bounds. When the sudden explosion occurs, my view on the timing and market size will change.
With this framework, let's look at each sub-field in detail.
2. Field 1: Decentralized Computing
Decentralized computing is the backbone of decentralized AI.
The GPU market, decentralized training, and decentralized inference are closely linked.
The supply side usually comes from small and medium-sized data centers and consumer GPUs.
The demand side is small but growing. Today, it comes from price-sensitive, latency-insensitive users and smaller AI startups.
The biggest challenge facing the Web3 GPU market right now is how to get them up and running.
Coordinating GPUs on a decentralized network requires advanced engineering and a well-designed, reliable network architecture.
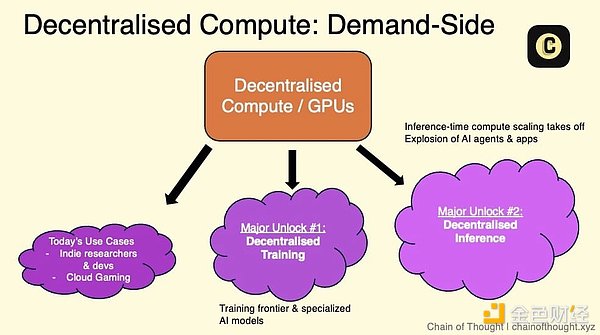
2.1 GPU Market/Computing Network
Several Crypto AI teams are building decentralized networks to leverage the world’s potential computing power in response to the GPU shortage that cannot meet demand.
The core value proposition of GPU marketplaces is threefold:
You can access compute at “90% less” than AWS because there are no middlemen and the supply side is open. Essentially, these marketplaces allow you to take advantage of the lowest marginal compute costs in the world.
Greater flexibility: no lock-in contracts, no KYC processes, no waiting times.
Censorship resistance
To address the supply side of the market, these markets source their computing power from:
Enterprise-grade GPUs (e.g. A100, H100) for small and medium data centers where demand is hard to find, or Bitcoin miners looking to diversify.
I also know of teams working on large government-funded infrastructure projects where data centers have been built as part of technology growth initiatives. These GPU providers are often incentivized to keep their GPUTs on the network, which helps them offset the amortized cost of the GPUs.
There are millions of consumer-grade GPUs for gamers and home users who connect their computers to the network in exchange for token rewards.
On the other hand, today’s demand for decentralized computing comes from:
Price-sensitive, latency-insensitive users. This market segment prioritizes price over speed. Think researchers exploring new areas, independent AI developers, and other cost-conscious users who don’t need real-time processing. Many of them may not be satisfied with traditional hyperscale servers like AWS or Azure due to budget constraints. Because they are widely distributed among the population, targeted marketing is critical to attract this group.
Small AI startups, who face the challenge of obtaining flexible, scalable compute resources without long-term contracts with major cloud providers. Business development is critical to attracting this segment as they actively seek alternatives to hyperscale lock-in.
Crypto AI startups, who build decentralized AI products but do not have their own computing power supply, will need to tap into the resources of one of these networks.
Cloud Gaming:While not directly AI-driven, cloud gaming is increasingly demanding GPU resources.
The key thing to remember is that developers always prioritize cost and reliability.
The real challenge is in demand, not supply.
Startups in this space often point to the size of their GPU supply network as a sign of success. But this is misleading - it is a vanity metric at best.
The real constraint is not supply, but demand. The key metric to track is not the number of GPUs available, but rather utilization and the number of GPUs actually rented out.
Tokens are great at bootstrapping supply, creating the incentives needed to scale quickly. However, they do not inherently solve the demand problem. The real test is getting the product to a state good enough that latent demand is realized.
Haseeb Qureshi (Dragonfly) said it well on this point:
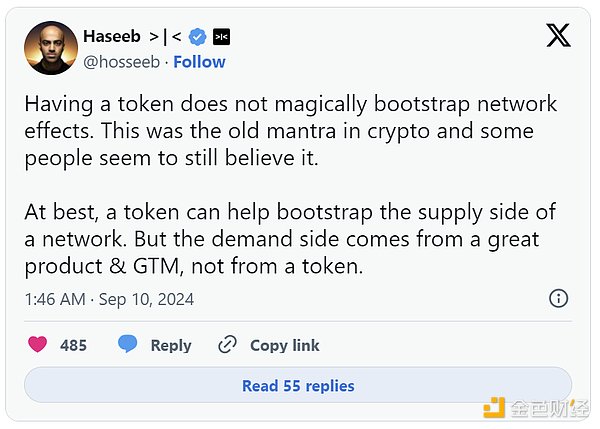
Making Compute Networks Actually Work
Contrary to popular belief, the biggest hurdle facing the web3 distributed GPU market right now is just getting them to work properly.
This is not a trivial problem.
Coordinating GPUs in a distributed network is incredibly complex, with so many challenges – resource allocation, dynamic workload scaling, load balancing across nodes and GPUs, latency management, data transfer, fault tolerance, and dealing with a wide variety of hardware spread across geographically dispersed locations. I could go on.
Achieving this requires thoughtful engineering and a solid, well-designed network architecture.
To put this into context, think about Google’s Kubernetes. It’s widely considered the gold standard for container orchestration, automating processes like load balancing and scaling in distributed environments, which are very similar to the challenges facing distributed GPU networks. Kubernetes itself is built on more than a decade of experience at Google, and even then it took years of relentless iteration to get it right.
Some of the GPU compute marketplaces that have come online today can handle small workloads, but have problems once they try to scale. I suspect this is because their architectural foundations are poorly designed.
Another challenge/opportunity for decentralized computing networks is ensuring trustworthiness: verifying that each node actually provides the computing power it claims. Currently, this relies on network reputation, and in some cases, computing power providers are ranked based on reputation scores. Blockchain seems to be well suited to a trustless verification system. Startups like Gensyn and Spheron are seeking to solve this problem using a trustless approach.
Today, many web3 teams are still working on these challenges, which also means that the door of opportunity is open.
Decentralized Computing Market Size
How big is the market for decentralized computing networks?
Today, it is probably only a small part of the cloud computing industry, which is worth $680 billion to $2.5 trillion. However, despite the increased friction for users, there will always be demand as long as the cost is lower than traditional providers.
I believe costs will remain low in the short to medium term due to token subsidies and supply unlocking for price-insensitive users (e.g. if I can rent out my gaming laptop to earn extra cash, whether it’s $20 or $50 per month, I’ll be happy).
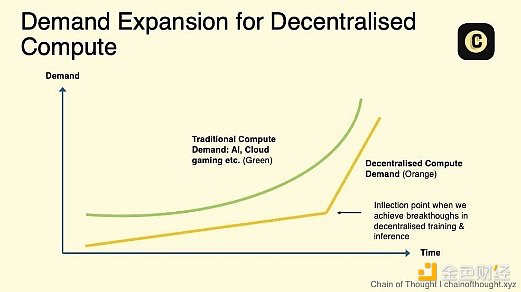
But the real growth potential of decentralized compute networks — and the true expansion of their TAM — will emerge when:
decentralized training of AI models becomes practical.
Demand for inference is surging and existing data centers can’t keep up. It’s already starting to show. Jensen Huang says inference demand will grow “a billion-fold.”
Adequate service level agreements (SLAs) are becoming available, addressing a key barrier to enterprise adoption. Currently, decentralized computing operates with varying levels of quality of service (e.g., uptime percentage). With SLAs, these networks can provide standardized reliability and performance metrics, making decentralized computing a viable alternative to traditional cloud computing providers.
Decentralized permissionless computing is the foundational layer of the decentralized AI ecosystem — the infrastructure.
While the supply chain for GPUs is expanding, I believe we are still at the dawn of the age of human intelligence. The demand for computing will be insatiable.
It is important to note that an inflection point that could trigger a re-rating of the entire GPU market may come soon.
Other notes:
The pure GPU market is crowded, with fierce competition between decentralized platforms and the rise of emerging cloud services for web2 AI such as Vast.ai and Lambda.
Small nodes (like 4 x H100) are not in great demand as their usage is limited, but good luck finding someone selling large clusters - they are still in some demand.
Will one dominant player aggregate all hashrate supply for decentralized protocols, or will it keep hashrate fragmented across multiple markets? I'm leaning towards the former, as consolidation generally leads to better infrastructure efficiency. But this will take time, and in the meantime, fragmentation and confusion continue.
Developers want to focus on application development rather than dealing with deployment and configuration. Marketplaces must abstract these complexities and make access to compute as frictionless as possible.
2.2 Decentralized Training
If the scaling law holds, then training the next generation of cutting-edge AI models in a single data center will one day become impossible.
Training AI models requires transferring large amounts of data between GPUs. The low data transfer (interconnection) speed between distributed GPUs is often the biggest obstacle.
Researchers are exploring multiple approaches simultaneously and are making breakthroughs (e.g. Open DiLoCo, DisTrO). These advances will add up and accelerate progress in the field.
With the move toward models like OpenAI o1, inference demand will soar, creating opportunities for decentralized inference networks.
Imagine this: a massive, world-changing AI model developed not in secret elite labs, but shaped by millions of ordinary people. Gamers whose GPUs normally create theatrical explosions of Call of Duty are now lending their hardware to something much bigger — an open-source, collectively owned AI model with no central gatekeeper.
In such a future, foundation-scale models aren’t just confined to top AI labs.
But let’s ground this vision in the present. Right now, the bulk of heavyweight AI training is still concentrated in centralized data centers, and this may be the norm for some time.
Companies like OpenAI are expanding their massive clusters. Elon Musk recently announced that xAI is about to build a datacenter with the equivalent of 200,000 H100 GPUs.
But it's not just about raw GPU counts. Model FLOPS Utilization (MFU), a metric proposed by Google in its 2022 PaLM research paper, tracks how efficiently a GPU's maximum capacity is used. Surprisingly, MFU usually hovers between 35-40%.
Why so low? In accordance with Moore's Law, GPU performance has suddenly skyrocketed in the past few years, but improvements in network, memory, and storage have lagged significantly, forming a bottleneck. As a result, GPUs are often in a limited state, waiting for data.
Today's AI training is still highly centralized because of one word - efficiency.
Training large models depends on the following techniques:
Data parallelism: Splitting a dataset across multiple GPUs to perform operations in parallel, speeding up the training process.
Model parallelism: Distributing parts of a model across multiple GPUs to get around memory constraints.
These approaches require GPUs to constantly exchange data, making the speed of the interconnection—the rate at which data can be transferred across computers in a network—critical.
When the cost of training a cutting-edge AI model can exceed $1 billion, every efficiency gain counts.
With high-speed interconnects, centralized data centers are able to quickly transfer data between GPUs and save a lot of money on training time that decentralized settings can’t match.
Overcoming Slow Interconnect Speeds
If you talk to people working in the AI field, many will tell you that decentralized training simply doesn’t work.
In a decentralized setting, clusters of GPUs are not physically co-located, so transferring data between them is much slower and becomes a bottleneck. Training requires the GPUs to synchronize and exchange data at every step. The farther apart they are, the higher the latency. Higher latency means slower training and higher costs.
What might take days in a centralized data center might stretch to two weeks in a decentralized data center, which is also more expensive. It’s simply not feasible.
But that’s about to change.
The good news is that there has been a surge of research interest in distributed training. Researchers are exploring multiple approaches simultaneously, as evidenced by the large number of studies and published papers. These advances will add up and converge to accelerate progress in the field.
It’s also about testing in production, seeing how far we can push the boundaries.
Some decentralized training techniques already work on smaller models on slow interconnects. Now, cutting-edge research is pushing these approaches to larger models.
For example, Prime Intellect’s open source DiCoLo paper shows a practical approach that involves GPU “islands” that perform 500 local steps before syncing, slashing bandwidth requirements by 500x. What started as Google DeepMind research on small models has scaled to training models with 10 billion parameters in 11 months, and is now fully open source.
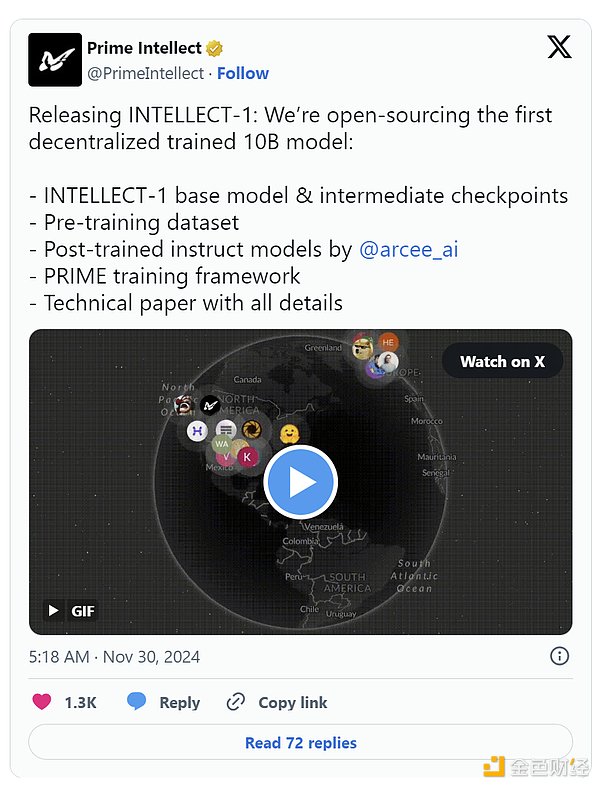
Nous Research is raising the bar with their DisTrO framework, which uses an optimizer to reduce inter-GPU communication requirements by an astounding 10,000x while training a 1.2B parameter model.
And the momentum is only growing. Last December, Nous announced pre-training of a 15B parameter model with loss curves (how the model error decreases over time) and convergence rates (how quickly the model performance stabilizes) that match or even beat typical results from centralized training. Yes, better than centralized.
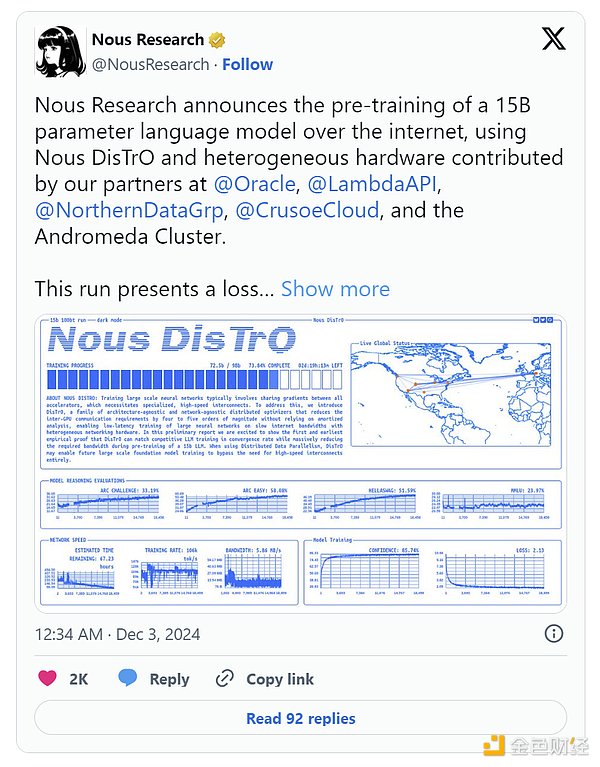
Managing the diverse range of GPU hardware is another big challenge, including the memory-constrained consumer GPUs typical of decentralized networks. Techniques like model parallelism (partitioning model layers across devices) can help achieve this.
The Future of Decentralized Training
Current decentralized training approaches still have model sizes far below cutting-edge models (GPT-4 reportedly has nearly a trillion parameters, 100x larger than Prime Intellect’s 10B model). To achieve true scale, we’ll need breakthroughs in model architectures, better network infrastructure, and smarter task distribution across devices.
We can dream big. Imagine a world where decentralized training can aggregate more GPU compute power than even the largest centralized data centers can muster.
Pluralis Research (a crack team focused on decentralized training that’s worth keeping an eye on) argues that this is not only possible, but inevitable. Centralized data centers are limited by physical constraints like space and power availability, while decentralized networks can tap into a truly unlimited global pool of resources.
Even NVIDIA’s Jensen Huang admits that asynchronous decentralized training could unlock the true potential of AI scaling. Distributed training networks are also more fault-tolerant.
So in one possible future world, the world’s most powerful AI models will be trained in a decentralized fashion.
It’s an exciting prospect, but I’m not completely convinced just yet. We need stronger evidence that decentralized training of the largest models is both technically and economically feasible.
I see great promise in this: the best of decentralized training may lie in small, specialized open source models designed for targeted use cases, rather than competing with super-large AGI-driven cutting-edge models. Certain architectures, especially non-transformer models, have proven to be well suited to decentralized settings.
There is another piece to the puzzle: tokens. Once decentralized training becomes feasible at scale, tokens can play a critical role in incentivizing and rewarding contributors, effectively bootstrapping these networks.
The road to this vision is long, but progress is encouraging. As future models scale beyond the capacity of a single datacenter, advances in decentralized training will benefit everyone, even large tech companies and top AI research labs.
The future is distributed. When a technology has such broad potential, history shows it always works better and faster than anyone expects.
2.3. Decentralized Reasoning
Currently, most of the computing power for AI is focused on training large-scale models. There is a race going on among the top AI labs to see who can develop the best underlying model and ultimately achieve AGI.
But my take is this: In the coming years, this focus on training will shift to reasoning. As AI becomes increasingly integrated into the applications we use every day—from healthcare to entertainment—the amount of computing resources required to support reasoning will be staggering.
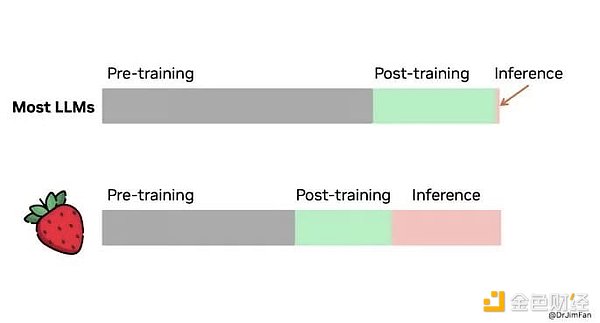
This is not just speculation. Inference-time compute scaling is the latest buzzword in AI. OpenAI recently released a preview/mini version of its latest model, 01 (codename: Strawberry). Is this a major shift? Take the time to think, first ask yourself what steps you should take to answer the question, and then work your way through them.
This model is designed for more complex, planning-intensive tasks, like crossword puzzles, and questions that require deeper reasoning. You’ll notice that it’s slower and takes more time to generate a response, but the results are more thoughtful and nuanced. It’s also much more expensive to run (25 times more than GPT-4).
The shift in focus is clear: the next leap in AI performance will come not just from training larger models, but from scaling up the application of compute during inference.
If you want to learn more, some research articles show that:
Once powerful models are trained, their inference tasks — what the models do — can be offloaded to decentralized computing networks. This makes sense because:
Inference requires far fewer resources than training.After training, models can be compressed and optimized using techniques such as quantization, pruning, or distillation. They can even be broken down to run on everyday consumer devices. You don’t need a high-end GPU to power inference.
This is already happening. Exo Labs has figured out how to run a 450B parameter Llama3 model on consumer hardware like MacBooks and Mac Minis. Distributing inference across multiple devices can handle large-scale workloads efficiently and cost-effectively.
Better user experience. Running computation closer to the user reduces latency, which is critical for real-time applications like gaming, AR, or self-driving cars. Every millisecond counts.
Think of decentralized inference as a CDN (content distribution network) for AI: Instead of connecting to nearby servers to quickly serve up websites, decentralized inference leverages local computing power to deliver AI responses in record time. By adopting decentralized inference, AI applications become more efficient, more responsive, and more reliable.
The trend is clear. Apple’s new M4 Pro chip competes with Nvidia’s RTX 3070 Ti, which until recently was the domain of hardcore gamers. Our hardware is increasingly capable of handling advanced AI workloads.
The Value Added in Crypto
For decentralized inference networks to succeed, there must be compelling economic incentives. Nodes in the network need to be compensated for their computing power contributions. The system must ensure that rewards are distributed fairly and efficiently. Geographic diversity is necessary to reduce latency in inference tasks and improve fault tolerance.
What is the best way to build a decentralized network? Crypto.
Tokens provide a powerful mechanism to align the interests of participants, ensuring that everyone is working towards the same goal: expanding the network and increasing the value of the token.
Tokens also accelerate the growth of the network. They help solve the classic chicken-and-egg problem that has held back most networks by rewarding early adopters and driving participation from day one.
The success of Bitcoin and Ethereum proves this - they have gathered the largest pools of computing power on the planet.
Decentralized inference networks will be next. With geographical diversity, they reduce latency, improve fault tolerance, and bring AI closer to users. With cryptographic incentives, they will scale faster and better than traditional networks.
(To be continued, stay tuned)